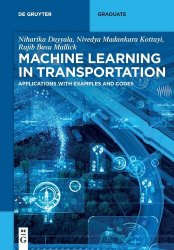
Автор: Niharika Dayyala, Nivedya Madankara Kottayi, Rajib Basu Mallick
Издательство: De Gruyter
Год: 2025
Страниц: 172
Язык: английский
Формат: pdf (true), epub
Размер: 47.7 MB
The book introduces the reader to Machine Learning in transportation. It discusses both simple and advanced concepts including core statistics, data wrangling, data visualization, supervised and unsupervised datamining techniques as well as text mining. The book prepares students to manage data, visualize data and apply appropriate Machine Learning techniques on transportation datasets to derive important insights. - State-of-the-art techniques in Machine Learning; - Applications in transportation engineering; - Includes MATLAB and Python codes. Deep Learning is a subset of Machine Learning, a subset of Artificial Intelligence (AI). In Deep Learning, the applicable concept is that the algorithm extracts the features based on which it can classify or regress, rather than the user having to specify them. The goal of the ANN is to output a function that matches the output, as given by the training data.