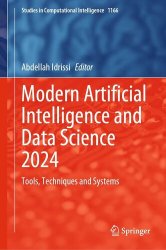
Автор: Abdellah Idrissi
Издательство: Springer
Серия: Studies in Computational Intelligence
Год: 2024
Страниц: 591
Язык: английский
Формат: pdf (true)
Размер: 21.0 MB
This book, through its various chapters presenting recent advances in Modern Artificial Intelligence and Data Science as well as their applications, aims to set up lasting and real applications necessary for both academics and professionals. The field of Data Science, for its part, has seen rapid growth in recent years, and thanks to the advances of AI, this growth has accelerated further. Artificial Intelligence and Data Science are two distinct fields, but they complement each other so well that their combination has become a powerful force for driving innovation and progress across all industries. Data Science provides the tools and techniques necessary to ensure that the data used to train AI systems is reliable, accurate and free of bias. This is more than essential to develop reliable AI systems that can make fair and unbiased decisions. It provides the methods, techniques and tools needed to collect, store, clean and analyze data to identify patterns and extract insights. In Part I, entitled Artificial Intelligence, Machine Learning and Deep Learning, the reader will discover everything that revolves around Machine Learning and Deep Learning. Machine learning, which is a common component of AI and Data Science, encompasses the development of algorithms and systems capable of learning from data, making predictions and/or decisions based on this data and also improve over time.