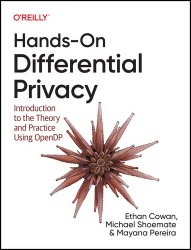
Автор: Ethan Cowan, Michael Shoemate, Mayana Pereira
Издательство: O’Reilly Media, Inc.
Год: 2024
Страниц: 362
Язык: английский
Формат: True PDF, True/Retail EPUB
Размер: 12.6 MB
Many organizations today analyze and share large, sensitive datasets about individuals. Whether these datasets cover healthcare details, financial records, or exam scores, it's become more difficult for organizations to protect an individual's information through deidentification, anonymization, and other traditional statistical disclosure limitation techniques. This practical book explains how differential privacy (DP) can help. Authors Ethan Cowan, Michael Shoemate, and Mayana Pereira explain how these techniques enable data scientists, researchers, and programmers to run statistical analyses that hide the contribution of any single individual. You'll dive into basic DP concepts and understand how to use open source tools to create differentially private statistics, explore how to assess the utility/privacy trade-offs, and learn how to integrate differential privacy into workflows. The OpenDP Library is part of the larger OpenDP Project. OpenDP is a community effort to build a trustworthy suite of open-source tools for enabling privacy-protective analysis of sensitive personal data, focused on a library of algorithms for generating differentially private statistical releases. The target use cases for OpenDP are to enable government, industry, and academic institutions to safely and confidently share sensitive data to support scientifically oriented research and exploration in the public interest. We aim for OpenDP to flexibly grow with the rapidly advancing science of differential privacy, and be a pathway to bring the newest algorithmic developments to a wide array of practitioners. SmartNoise is a collaboration between Microsoft, Harvard’s Institute for Quantitative Social Science (IQSS), and the OpenDP Project. The project aims to connect solutions from the research community with the lessons learned from real-world deployments to make differential privacy broadly accessible. Building upon the foundation of the OpenDP Library, the SmartNoise SDK includes two Python packages: smartnoise-sql and smartnoise-synth.