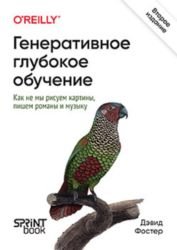
Название: Генеративное глубокое обучение. Как не мы рисуем картины, пишем романы и музыку, 2-е межд. издание
Автор: Дэвид Фостер
Издательство: Спринт Бук
Год: 2024
Формат: pdf
Страниц: 448
Размер: 18,2 Мб
Язык: русский
Генеративный ИИ — одна из самых обсуждаемых тем в сфере технологий. Пора разобраться с возможностями TensorFlow и Keras, чтобы с легкостью создавать впечатляющие генеративные модели глубокого обучения, включая вариационные автокодировщики (VAE), генеративно-состязательные сети (GAN), трансформеры, нормализующие потоки, модели на основе энергии и диффузионные модели удаления шума. Дэвид Фостер, начинает с основ глубокого обучения и постепенно переходит к передовым архитектурам. Благодаря его советам и подсказкам вы узнаете, как повысить эффективность обучения и творческие возможности ваших моделей. Книга была полностью обновлена и переработана, чтобы соответствовать текущему развитию генеративного обучения.