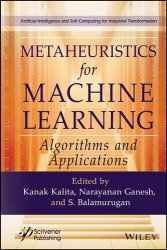
Автор: Kanak Kalita, Narayanan Ganesh
Издательство: Wiley-Scrivener
Серия: Artificial Intelligence and Soft Computing for Industrial Transformation
Год: 2024
Страниц: 327
Язык: английский
Формат: pdf, epub
Размер: 10.1 MB
The book unlocks the power of nature-inspired optimization in Machine Learning and presents a comprehensive guide to cutting-edge algorithms, interdisciplinary insights, and real-world applications. The field of metaheuristic optimization algorithms is experiencing rapid growth, both in academic research and industrial applications. These nature-inspired algorithms, which draw on phenomena like evolution, swarm behavior, and neural systems, have shown remarkable efficiency in solving complex optimization problems. With advancements in Machine Learning and Artificial Intelligence, the application of metaheuristic optimization techniques has expanded, demonstrating significant potential in optimizing Machine Learning models, hyperparameter tuning, and feature selection, among other use-cases. In the industrial landscape, these techniques are becoming indispensable for solving real-world problems in sectors ranging from healthcare to cybersecurity and sustainability. Businesses are incorporating metaheuristic optimization into Machine Learning workflows to improve decision-making, automate processes, and enhance system performance. As the boundaries of what is computationally possible continue to expand, the integration of metaheuristic optimization and Machine Learning represents a pioneering frontier in computational intelligence, making this book a timely resource for anyone involved in this interdisciplinary field. Genetic algorithms (GAs) fit to a cluster of metaheuristic optimization techniques that draw inspiration from natural selection and genetics. In order to find the optimal solution for a particular issue, the core idea underlying the GA is to mimic the evolutionary process.