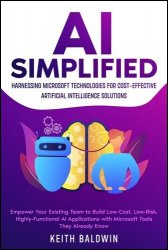
Автор: Keith Baldwin
Издательство: eBookIt.com
Год: 2024
Страниц: 223
Язык: английский
Формат: pdf (true)
Размер: 23.2 MB
AI Simplified: Harnessing Microsoft Technologies for Cost-Effective Artificial Intelligence Solutions is your practical guide to unlocking the power of AI within your organization without breaking the bank or hiring new specialized teams. Tailored for business leaders, executives, managers, and technical professionals, this book demystifies Artificial Intelligence (AI) and provides actionable steps to integrate AI solutions effectively using tools and platforms you already know. The main goal of this book is to equip business leaders, executives, managers, programmers, and database administrators with the knowledge and tools they need to effectively leverage Artificial Intelligence (AI) within their organizations. Our mission is to make AI approachable and actionable for businesses eager to exploit this potential. AI can appear overwhelming, shrouded in jargon and complex technicalities. Yet, when demystified and implemented effectively, it can address critical business challenges, automate mundane tasks, perform functions beyond the scope of traditional programming, and provide insights that inform strategic decisions.