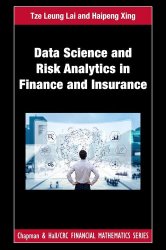
Автор: Tze Leung Lai, Haipeng Xing
Издательство: CRC Press
Год: 2025
Страниц: 464
Язык: английский
Формат: pdf (true)
Размер: 10.1 MB
This book presents statistics and Data Science methods for risk analytics in quantitative finance and insurance. Part I covers the background, financial models, and data analytical methods for market risk, credit risk, and operational risk in financial instruments, as well as models of risk premium and insolvency in insurance contracts. Part II provides an overview of Machine Learning (including supervised, unsupervised, and reinforcement learning), Monte Carlo simulation, and sequential analysis techniques for risk analytics. In Part III, the book offers a non-technical introduction to four key areas in financial technology: Artificial Intelligence, blockchain, cloud computing, and big data analytics. In general, machine learning methods are broadly categorized into three main types: supervised learning, unsupervised learning, and reinforcement learning. This chapter provides an overview of some commonly used supervised and unsupervised methods and explores their applications in finance. The subsequent chapter presents Markov decision processes and widely used reinforcement learning techniques, further expanding the understanding of machine learning’s role in optimizing decision-making processes within the financial domain.