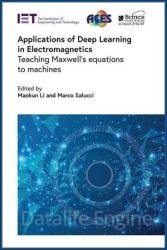
Автор: Maokun Li, Marco Salucci
Издательство: Scitech Publishing
Год: 2023
Страниц: 480
Язык: английский
Формат: pdf (true)
Размер: 31.9 MB
Deep Learning has started to be applied to solving many electromagnetic problems, including the development of fast modelling solvers, accurate imaging algorithms, efficient design tools for antennas, as well as tools for wireless links/channels characterization. The contents of this book represent pioneer applications of Deep Learning techniques to electromagnetic engineering, where physical principles described by the Maxwell's equations dominate. With the development of Deep Learning techniques, improvement in learning capacity and generalization ability may allow machines to "learn" from properly collected data and "master" the physical laws in certain controlled boundary conditions. In the long run, a hybridization of fundamental physical principles with knowledge from training data could unleash numerous possibilities in electromagnetic theory and engineering that used to be impossible due to the limit of data information and ability of computation.
Electromagnetic applications of Deep Learning covered in the book include electromagnetic forward modeling, free-space inverse scattering, non-destructive testing and evaluation, subsurface imaging, biomedical imaging, direction of arrival estimation, remote sensing, digital satellite communications, imaging and gesture recognition, metamaterials and metasurfaces design, as well as microwave circuit modeling.
With the help of big data, massive parallelization, and computational algorithms, Deep Learning (DL) techniques have been developed rapidly during the recent years. Complex artificial neural networks, trained by large amounts of data, have demonstrated unprecedented performance in many tasks in artificial intelligence, such as image and speech recognition. This success also leads DL into many other fields of engineering. And electromagnetics (EM) is one of them.
This book is intended to overview the recent research progresses in applying DL techniques in EM engineering. Traditionally, research and development in this field have been always based on EM theory. The EM field distribution in engineering problems is modeled and solved by means of Maxwell’s equations. The results can be very accurate, especially with the help of modern computational tools. However, when the system gets more complex, it is tough to solve because the increase in the degree-of-freedom exceeds the modeling and computational capabilities. Meanwhile, the demand for real-time computing also poses a significant challenge in the current EM modeling procedure.
DL can be used to alleviate some of the above challenges. First, it can “learn” from measured data and master some information about the complex scenarios for the solution procedure, which can improve the accuracy of modeling and data processing. Second, it can reduce the computational complexity in EM modeling by building fast surrogate models. Third, it can discover new designs and accelerate the design process while combining with other design tools. More engineering applications are being investigated with deep learning techniques, such as antenna design, circuit modeling, EM sensing and imaging, etc. The contents of the book are as follows:
In Chapter 1, a brief introduction to machine learning with a focus on DL is discussed. Basic concepts and taxonomy are presented. The classification of DL techniques is summarized, including supervised learning, unsupervised learning, and reinforcement learning. Moreover, popular DL architectures such as convolutional neural networks, recurrent neural networks, generative adversarial networks, and auto-encoders are presented.
Chapter 2 reviews the recent advances in DL techniques as applied to EM forward modeling. Traditional EM modeling uses numerical algorithms to solve Maxwell’s equations, such as the method of moments, the finite element method, and the finite difference time domain method. In this context, DL can establish the mapping between a physical model and the corresponding field distribution. In other words, it is possible to predict the field distribution without solving partial differential equations, resulting in a much faster computation speed.
...
Chapter 12 describes DL as applied to microwave circuit modeling, an important area of computer-aided design for fast and accurate microwave design and optimization.The feed-forward deep neural network and the vanishing gradient problem during its training process are introduced. Various recurrent neural networks for nonlinear circuit modeling are presented. Several application examples are presented to demonstrate the capabilities of deep neural network modeling techniques. As widely demonstrated through the Chapter, the powerful learning ability of DL makes it a suitable choice for modeling the complex input-output relationship of microwave circuits.
Based on these discussions, Chapter 13 summarizes the pros and cons of DL when applied to EM engineering, envisaging challenges and future trends in this area, and drawing some concluding remarks.
Applications of Deep Learning in Electromagnetics contains valuable information for researchers looking for new tools to solve Maxwell's equations, students of electromagnetic theory, and researchers in the field of Deep Learning with an interest in novel applications.
Скачать Applications of Deep Learning in Electromagnetics: Teaching Maxwell's equations to machines

[related-news] [/related-news]
Комментарии 0
Комментариев пока нет. Стань первым!