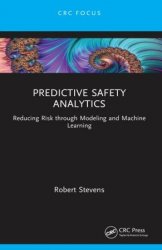
Автор: Robert Stevens
Издательство: CRC Press
Год: 2024
Страниц: 99
Язык: английский
Формат: pdf (true), djvu
Размер: 10.2 MB
Nearly all our safety data collection and reporting systems are backwardlooking: incident reports; dashboards; compliance monitoring systems; and so on. This book shows how we can use safety data in a forward-looking, predictive sense.
Predictive Safety Analytics: Reducing Risk through Modeling and Machine Learning contains real use cases where organizations have reduced incidents by employing predictive analytics to foresee and mitigate future risks. It discusses how Predictive Safety Analytics is an opportunity to break through the plateau problem where safety rate improvements have stagnated in many organizations. The book presents how the use of data, coupled with advanced analytical techniques, including machine learning, has become a proven and successful innovation. Emphasis is placed on how the book can “meet you where you are” by illuminating a path to get there, starting with simple data the organization likely already has. Highlights of the book are the real examples and case studies that will assist in generating thoughts and ideas for what might work for individual readers and how they can adapt the information to their particular situations.
Data scientists speak of their workflow in terms of a pipeline. In fact, many modern software tools for data science build upon this concept to manage the tasks associated with building models. There is a myriad of ways pipelines are put forth, and no one scheme rules them all. The steps in the pipeline flow are the following:
Source data includes a wide variety of data sources, with varying content and in different formats. Examples of these are discussed further in the chapter titled, The Safety Data Repository. The data can come directly from an existing safety management system (SMS) or other operational databases. It may also include third-party and external data. The formats can include formal database structures such as SQL Server, Excel files, or flat (e.g., comma-segmented values) files. The data engineer has tools to extract and load data from each of these sources.
Import and process is also known as data ingestion and integration, or extract, transform, and load (ETL). Sometimes the euphemisms “data munging” or “data wrangling” are used. A part of this step includes reporting on data anomalies. Most data contain flaws in that there are missing values, extreme values, or infeasible values.
Merge is where the data is combined. Data sets are joined using key variables. For example, an employee ID number in an incident reporting system would be used to join with human resource data about the employee using that same ID number as the key.
Feature Engineering is the phrase data scientists use to describe special transformations and derivatives of the variables in the raw data. The Machine Learning community uses the term “feature” to mean the same thing that the statistics community calls an “independent variable”. A database professional may simply call them “columns”.
Python is a broad programming language with strengths in manipulating data. A large variety of “libraries” are available that are purpose-driven, such as implementing a specific Machine Learning algorithm or a collection of algorithms. Related to libraries, and often synonymous with them are “frameworks”. These work as add-ins or wrappers around Python to do modeling. Some of the more common ones are Pandas, Scikit-Learn, Numpy, Tensorflow, Keras, PyTorch, Theano, and LightGBM. This ecosystem of tools rapidly evolves. Python has emerged in recent years as the toolset of choice for data scientists.
This book is written for professionals and researchers in system reliability, risk and safety assessment, quality control, operational managers in selected industries, data scientists, and Machine Learning engineers. Students taking courses in these areas will also find this book of interest to them.
Скачать Predictive Safety Analytics: Reducing Risk through Modeling and Machine Learning

[related-news] [/related-news]
Комментарии 0
Комментариев пока нет. Стань первым!