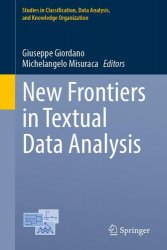
Автор: Giuseppe Giordano, Michelangelo Misuraca
Издательство: Springer
Год: 2024
Страниц: 385
Язык: английский
Формат: pdf (true), epub
Размер: 39.4 MB
This volume presents a selection of articles which explore methodological and applicative aspects of textual data analysis. Divided into four parts, it begins by focusing on statistical methods, and then moves on to problems in quantitative language processing. After discussing the challenging task of text mining in relation to emotional and sentiment analyses, the book concludes with a collection of studies in the social sciences and public health which apply textual data analysis methods.
The book, comprising thirty contributions, is divided into four parts: (1). Statistical methods for Textual Data Analysis, (2). Advances in language processing, (3). Emotion and Sentiment Analyses, and (4). Textual Data Analysis in action.
Machine learning has become a crucial technology in various industries and has many applications ranging from computer vision and natural language processing to predictive policing and healthcare. Artificial Intelligence (AI) models can be grouped into several families based on their underlying architecture, functionality, and applications. The most important ones are the following:
• 1. Deep Learning models: They have been the driving force behind many breakthroughs in AI in the recent year. Their architecture, made of multiple neuronal layers, is designed to model complex relationships and nonlinear interactions between input and output variables.
• 2. Generative models: They can generate new data much of a muchness to the fed training data. They are used in most of applications generating new images, music, or text.
• 3. Reinforcement Learning models: They can learn from interactions with their environment and make decisions based on their experience. This has the potential to be applied to a wide range of real-world problems, such as autonomous systems and robotics.
• 4. Transfer learning models: They allow for efficient and effective training by transferring knowledge from one task to another related task. This can be a cost-effective solution for many real-world computer vision and NLP applications.
Many generative, reinforcement learning and transfer learning models rely on deep neural network architectures. Indeed, generative models, such as GANs and VAEs, use multiple layers of neural networks to learn the underlying patterns and relationships in the data. Also, reinforcement learning models such as Deep Q-Network (DQN), policy gradients, A3C (Asynchronous Advantage Actor-Critic), and proximal policy optimization (PPO) use deep neural network architectures to learn from interactions with their environment and make decisions based on their experience. Also, state-of-the-art transfer learning models such as VGG, ResNet, BERT, and GPT-2/3 make use of deep neural architectures to transfer knowledge from one task to another related one.
Despite the wide range of deep neural network applications, they are considered to be black box models as they do not provide explicit and intuitive explanations for their predictions. One bridge toward complex Deep Learning explainability is to mix those models with classic approaches. Even though the classic model to mix with is a black box, such as SVM, explaining their results remains easier than deep neural networks. Moreover, recent research has been focusing on developing methods to make SVM more interpretable, such as visualizing the decision boundary or incorporating domain knowledge into the model to help explain predictions. Once the explainability is met, the hybrid model’s main challenge is maintaining its discriminative power. For this purpose, we perform statistical profiling of deep neural networks’ effectiveness using a hybrid model of convolutional neural networks and support vector machines as a use case.
Up until now, in the field of Natural Language Processing (NLP) and Computational Text Analysis Methods (CTAM) most studies focused on logical-grammatical analysis or, more recently, on content and sentiment analysis. However, there is still limited reference to the role of the discursive process: that is, how language’s use shapes the reality of sense in which we live in. But how can we gain a deep knowledge and understanding of the sense of what is conveyed by a text? In order to investigate the process of sense’s reality configuration, we introduce Dialogic Process Analysis. Starting from the formalization of 24 rules of natural language’s use of transversal to every idiom, called Discursive Repertories, Dialogic Process Analysis allows to describe how discursive processes unravel and to trace precisely the elements that generate each specific sense’s reality, which may be different even when contents and meanings are the same. Although researchers are able to denominate the Discursive Repertories, performing such a task requires specific and complex analysis expertise: that is why the application of Machine Learning models can lighten these problems. Thus, in this work we present the Dialogic Process Analysis research programme, its experimentations and results in the definition of its own Machine Learning model for textual data analysis and its future lines of development.
Скачать New Frontiers in Textual Data Analysis

[related-news] [/related-news]
Комментарии 0
Комментариев пока нет. Стань первым!