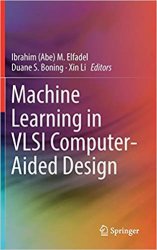
Автор: Ibrahim (Abe) M. Elfadel, Duane S. Boning, Xin Li
Издательство: Springer
ISBN: 3030046656
Год: 2019
Страниц: 697
Язык: английский
Формат: pdf (true), epub
Размер: 115.6 MB
This book provides readers with an up-to-date account of the use of machine learning frameworks, methodologies, algorithms and techniques in the context of computer-aided design (CAD) for very-large-scale integrated circuits (VLSI). Coverage includes the various machine learning methods used in lithography, physical design, yield prediction, post-silicon performance analysis, reliability and failure analysis, power and thermal analysis, analog design, logic synthesis, verification, and neuromorphic design.
Provides up-to-date information on machine learning in VLSI CAD for device modeling, layout verifications, yield prediction, post-silicon validation, and reliability;
Discusses the use of machine learning techniques in the context of analog and digital synthesis;
Demonstrates how to formulate VLSI CAD objectives as machine learning problems and provides a comprehensive treatment of their efficient solutions;
Discusses the tradeoff between the cost of collecting data and prediction accuracy and provides a methodology for using prior data to reduce cost of data collection in the design, testing and validation of both analog and digital VLSI designs.
As an active branch of applied computer science, the field of VLSI computer-aided design (VLSI CAD) has always been at the technological forefront in incorporating cutting-edge algorithms in the software tools and methodologies that electronics engineers have used to weave the digital fabric of our world.
This book amply demonstrates that in line with its historical track record, VLSI CAD has also been at the leading edge in making good use of machine-learning technologies to further automate the design, verification, and implementation of the most advanced chips. Machine learning and VLSI CAD have in common several main characteristics that may have greatly facilitated their interlock.
Contents:
Скачать Machine Learning in VLSI Computer-Aided Design

[related-news] [/related-news]
Комментарии 0
Комментариев пока нет. Стань первым!