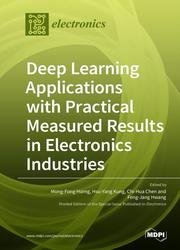
Автор: Mong-Fong Horng, Hsu-Yang Kung
Издательство: Mdpi AG
Год: 2020
Страниц: 274
Язык: английский
Формат: pdf (true)
Размер: 33.7 MB
Machine Learning (ML) and Deep Learning (DL) techniques have been the crucial tools when it comes to the feature extracting and event estimating for developing applications in the electronics industries. Some techniques have been implemented in the embedded systems and applied to industry 4.0 applications, industrial electronics applications, consumer electronics applications, and other electronics applications. For instance, supervised learning techniques, including neural networks (NN), convolutional neural networks (CNN), and recurrent neural networks (RNN), can be adopted for prediction applications and classification applications in the electronics industries.
Unsupervised learning techniques, including restricted Boltzmann machine (RBM), deep belief networks (DBN), deep Boltzmann machine (DBM), auto-encoders (AE), and denoising auto-encoders (DAE), can be used for denoising and generalization. Furthermore, reinforcement learning techniques, including generative adversarial networks (GANs) and deep Q-networks (DQNs), can be used to obtain generative networks and discriminative networks for contesting and optimizing in a zero-sum game framework. These techniques can provide the precise prediction and classification for electronics applications. Therefore, the aim of this Special Issue is to introduce the readers the state-of-the-art research work on Deep Learning applications with practical measured results in electronics industries.
This book collects 14 articles from the Special Issue entitled "Deep Learning Applications with Practical Measured Results in Electronics Industries" of Electronics. Topics covered in this Issue include four main parts: (1) environmental information analyses and predictions, (2) unmanned aerial vehicle (UAV) and object tracking applications, (3) measurement and denoising techniques, and (4) recommendation systems and education systems. These authors used and improved deep learning techniques (e.g., ResNet (deep residual network), Faster-RCNN (faster regions with convolutional neural network), LSTM (long short term memory), ConvLSTM (convolutional LSTM), GAN (generative adversarial network), etc.) to analyze and denoise measured data in a variety of applications and services (e.g., wind speed prediction, air quality prediction, underground mine applications, neural audio caption, etc.). Several practical experiments were conducted, and the results indicate that the performance of the presented deep learning methods is improved compared with the performance of conventional machine learning methods.
Скачать Deep Learning Applications with Practical Measured Results in Electronics Industries

[related-news] [/related-news]
Комментарии 0
Комментариев пока нет. Стань первым!