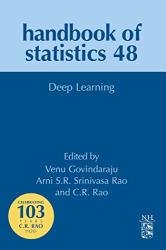
Автор: Venu Govindaraju, Arni S.R. Srinivasa Rao, C.R. Rao
Издательство: Academic Press/Elsevier
Год: 2023
Страниц: 270
Язык: английский
Формат: pdf (true)
Размер: 51.8 MB
Deep Learning, Volume 48 in the Handbook of Statistics series, highlights new advances in the field, with this new volume presenting interesting chapters on a variety of timely topics, including Generative Adversarial Networks for Biometric Synthesis, Data Science and Pattern Recognition, Facial Data Analysis, Deep Learning in Electronics, Pattern Recognition, Computer Vision and Image Processing, Mechanical Systems, Crop Technology and Weather, Manipulating Faces for Identity Theft via Morphing and Deepfake, Biomedical Engineering, and more.
Provides the authority and expertise of leading contributors from an international board of authors
Presents the latest release in the Handbook of Statistics series
Includes the latest information on Deep Learning
Over the last decade, the science of deep learning has seen rapid growth in terms of both theory and applicability. Statisticians, mathematicians, computer scientists, and other engineering and basic scientists have come together to design deep learning theories and experiments. One of the advantages of carefully planned deep learning experiments is the reduction of uncertainty in predictions and an increase in the performance of machines that are designed for a specific task. Through this volume of Handbook of Statistics on “deep learning,” we are aiming to illustrate the more realistic potential of the advancements made in the field of deep learning. We provide a wonderful collection of a wide range of research topics through didactic chapters.
This collection of chapters will be useful as starting points for serious graduate students and as summaries for senior researchers. The content ranges from foundations of deep learning models and probabilities of object detection to deep learning models that involve statistical techniques such as Bayesian, principal component analysis, regression approaches, etc. We have taken great effort to include practically implementable content such as facial recognition analysis and understanding the biases introduced in data, climate models, industrial applications, healthcare settings, and open challenges and ground-level difficulties faced in advanced applications in different settings.
The chapters in this volume are divided into the following three sections:
• Section I: Foundations
• Section II: Advanced Methods
• Section III: Transformative Applications
In Section I, Chapter 1 introduces new machines called exact Deep Learning machines and the concept of detecting an object by a machine with probability 1. Such machines are argued to be the best possible alternatives to the originally proposed AI models of the mid-1950s. Chapter 2 provides a well-written introduction and review of contextual embedding and related studies. The chapter also describes dimensionality reduction techniques using principal component analysis and preserving the information of the original data. Here, the recent advances made in novel contextual embedding are also detailed. Chapter 3 provides detailed foundations and training on Deep Learning principles in adversarial attacks and their wide range of applications. The chapter provides an excellent overview of the defense mechanisms to be adapted in adversarial attacks.
Section II comprises two chapters on advanced Deep Learning methods. Chapter 4 introduces advanced statistical methods and provides extremely clear insights into deep metrics, embedding spaces, and their utility in Computer Vision research. The chapter details the recent discussions on the design of experiments in computer vision studies and sampling strategies. The current state-of-the-art deep metric learning approaches described in the chapter provide training materials for researchers. Chapter 5 combines advanced methods of unsupervised domain adaptation in Computer Vision and image processing. Algorithms that transfer information to target domains and a comparison between single-trained source models and multiple-trained source models are introduced as well. The authors own algorithms for the effective aggregation of source models for nonuniform types of source data.
Section III comprises three chapters that describe the practical applications of Deep Learning methods in various real-world scenarios. Chapter 6 provides a detailed account of the successful implementation of various innovative projects conducted at different centers of the Council of Scientific and Industrial Research (CSIR) in India. These include climate prediction modeling, diagnosis of tumors, prediction of renewable energy generation, etc. The methods and designs of these experiments provide a training module for other such experiments. Chapter 7 summarizes the recent advancements in facial recognition analysis, data limitations, and associated statistical biases. Here, limitations in the current approaches and implementable newer methods to overcome the biases introduced due to a lack of enough data are described. The chapter concludes with open challenges and building blocks in facial analysis research worldwide. Chapter 8 discusses the latest advancements and highly implementable approaches in protecting against identity theft via morphing and deepfake of faces. Here, the fundamental techniques in morphing and deepfake and their advantages and disadvantages are outlined. The chapter reviews algorithms to protect against identity thefts, defense algorithms built to detect these manipulations, etc. It concludes with highly interesting open challenges associated with such research studies.
Contents:
Скачать Deep Learning: Handbook of Statistics, Volume 48

[related-news] [/related-news]
Комментарии 0
Комментариев пока нет. Стань первым!