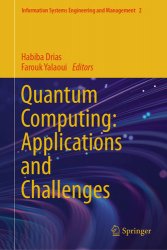
Автор: Habiba Drias, Farouk Yalaoui
Издательство: Springer
Год: 2024
Страниц: 226
Язык: английский
Формат: pdf (true), epub
Размер: 28.4 MB
This book is intended for academics, researchers, Ph.D. students, and industrials to learn about Quantum Computing and prepare them to understand the future with its cutting-edge tools. Quantum Computing holds great promise in terms of human life quality especially when combined with Artificial Intelligence (AI). In addition to Quantum Computing, the book focuses on Quantum Artificial Intelligence, quantum machine and Deep Learning, quantum cryptography, and quantum logistics optimization. Applications in weather forecasting, digital soil mapping, skin lesion classification, combinatorial optimization, and urgent transportation, among others, are presented.
Quantum computing promises exponentially faster computation and the ability to solve problems that are intractable by classical computers. This challenging field has the power to revolutionize cryptography, optimization, Artificial Intelligence, and simulations that could transform industries ranging from finance to drug discovery.
The opportunities of quantum technologies are enormous. The calculations carried out by quantum systems are very fast and their spin-offs in industry are very beneficial. This advance will be more talented by hybridizing it with artificial intelligence. Unlike this gainful progress for our society, quantum technologies unfortunately pose threats. The most striking example is the attack on cryptography. Computer security is highly threatened by quantum technologies.
Both opportunities and threats favor the emergence of start-ups, particularly in the fields of quantum computing, quantum communications, quantum post-cryptography and quantum artificial intelligence. Classical computing dealing with data will have to migrate to quantum computing and the sooner the better to ensure data protection.
Quantum machine learning (QML) is an interdisciplinary field combining quantum computing (QC) and machine learning (ML). It has gained increased attention due to advances in near-term hardware implementations of quantum devices. The use of QML has proven to result in a significant improvement in performance and computational speed. Consequently, QML has become an effective technique for data processing and classification.
Contents:
Скачать Quantum Computing: Applications and Challenges

[related-news] [/related-news]
Комментарии 0
Комментариев пока нет. Стань первым!