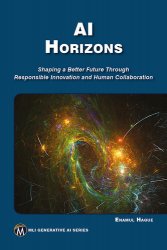
Автор: Enamul Haque
Издательство: Mercury Learning and Information
Год: 2024
Страниц: 245
Язык: английский
Формат: pdf (true), epub
Размер: 10.1 MB
As we stand on the brink of a new era, this book offers a comprehensive exploration of AI's ethical, social, and technological implications. It explores privacy, fairness, accountability, and the digital divide. The book features real-world case studies across diverse industries to illustrate AI's transformative power and its potential to revolutionize healthcare, education, transportation, and more. Special attention is given to the adoption of AI in emerging markets and emphasizes the importance of human-AI collaboration, where AI augments human intelligence and creativity rather than replacing it. It examines the need for human-centric design while also exploring AI governance and the role of multi-stakeholder collaboration in shaping the future of this technology. By fostering a comprehensive understanding of AI and its myriad implications, the book inspires thoughtful dialogue and responsible action to ensure a brighter, more equitable future for all. It is intended for business leaders, policymakers, academics, and curious individuals eager to understand AI’s role in shaping our world and how we can responsibly harness its power.
Machine Learning (ML) represents a specialized branch within the broader field of artificial intelligence, focusing primarily on crafting computer programs capable of learning and evolving through experience. At its heart, ML revolves around developing algorithms designed to sift through and analyze extensive datasets, discerning patterns within. This process empowers these systems to make informed predictions or decisions based on their analyses.
Crucially, ML algorithms are broadly classified into three categories: supervised learning, unsupervised learning, and reinforcement learning. Each type represents a unique method for training these algorithms, each with its distinct set of uses and inherent limitations. Supervised learning involves training the model on a labeled dataset, teaching it to recognize patterns and make predictions. Unsupervised learning, in contrast, deals with unlabeled data, allowing the algorithm to identify structures and patterns on its own. Reinforcement learning is a dynamic process where algorithms learn to make decisions by performing actions and observing the results or feedback from those actions. Each of these learning types unlocks different capabilities and applications in machine learning.
Deep Learning (DL), a specialized area within machine learning, uses what we call “neural networks” that have many layers, making them “deep.” Imagine trying to mimic how our brains work; with all their complex connections, that is what these networks attempt to do. They learn from enormous amounts of data. A neural network with just one layer can do some basic guesswork, much making a rough sketch. As you add more layers, the picture gets clearer and more detailed. These extra layers help the machine be more accurate and identify complicated patterns. It is like having a team where each member looks at a problem from a different angle and figures it out better.
Generative AI (GenAI), controlled by large language models, is like a digital artist capable of producing original pieces, whether it is music, art, or blocks of text. These models are not just replicating; they are inventing, using their training to fabricate new data that mirrors the examples they have learned from. An example in this field is ChatGPT from OpenAI, renowned for its ability to generate strikingly human-like text based on the prompts it receives. We will examine the mechanics and implications of generative AI, including a simpler breakdown of how it functions, in Chapter 8 of this book.
Large Language Models (LLMs) are neural networks fed with an enormous amount of text data, and they are remarkable for their language processing ability. LLMs are trained to digest this wealth of information and then generate text that appears human-like. You have likely encountered them in the form of chatbots or content creation tools.
Artificial Intelligence affects many industries, transforming sectors and reshaping decision-making. How can we trust decisions made by machines if we do not understand how they arrived at them? The answer to this puzzle lies in the domain of Explainable AI, often called XAI. Imagine for a moment a seasoned physician presented with a diagnosis from a new AI tool. The tool claims that a patient, based on numerous variables and data points, has a certain medical condition. The doctor wonders, “Why? What did the AI see or interpret that led to this conclusion?” It is insufficient for the doctor (or the patient) to merely accept the AI’s assertion; they need an explanation, a rationale. That is where XAI becomes important, as bridges the confusing Machine Learning algorithms and the clarity humans need. Instead of black-box decisions where outcomes emerge without insight into the process, XAI shows the inner workings of these complex systems. It enables AI to articulate its decision-making process in terms humans can understand, leveraging visualizations, straightforward language, or comparative data points.
Features:
Emphasizes human-AI symbiosis, highlighting human-centric design and responsible development
Provides real-world examples across industries, showcasing AI's transformative impact
Explores AI adoption in emerging economies, addressing unique challenges and opportunities
Table of contents:
1: Focusing on AI Ethics and Social Impact. 2. AI Applications and Real-World Case Studies. 3: AI in Emerging Markets.
4: The Human-AI Partnership. 5: AI Governance and Policy. 6: Public Perception, Acceptance, and Literacy of AI.
7: AI Risk Factors and How to Mitigate Them. 8: Generative AI: Conversational Agents and Beyond. References. Index.
Скачать AI Horizons: Shaping a Better Future Through Responsible Innovation and Human Collaboration

[related-news] [/related-news]
Комментарии 0
Комментариев пока нет. Стань первым!