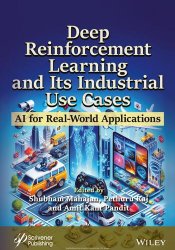
Автор: Shubham Mahajan, Pethuru Raj, Amit Kant Pandit
Издательство: Wiley-Scrivener
Год: 2025
Страниц: 403
Язык: английский
Формат: pdf (true)
Размер: 46.6 MB
“Deep Reinforcement Learning and its Industrial Use Cases: Harnessing AI for Real-World Applications” is an essential guide that supplies complex theories, practical insights, and diverse case studies behind deep reinforcement learning. This book offers a comprehensive look into how DLR is revolutionizing fields by implementing advanced algorithms in a variety of industries to solve real-world problems. Beyond the realm of successes of DLR, it critically examines challenges, pitfalls, and ethical considerations. This research and knowledge explore insights to meet the needs of curious enthusiasts eager to understand the cutting-edge technology shaping our future.
Throughout the pages of this book, we seek to discover the inner workings of DLR and its real-world applications. We start by laying down the foundational principles of reinforcement learning and building up to advanced DLR algorithms and techniques. Along the way, we delve into diverse case studies, examining how leading organizations harness the power of DLR to drive innovation and gain a competitive edge. From financial trading to autonomous manufacturing systems, each case study offers valuable insights into the practical considerations and challenges involved in deploying DLR solutions.
Deep reinforcement learning is a Machine Learning subfield that deals with teaching agents to make successive decisions in a given environment in order to maximize a cumulative reward. DRL algorithms approximation rules or function values with neural networks, allowing agents to learn sophisticated behaviors directly from raw sensory input.
Deep reinforcement learning (DRL) hybrid approaches relate to the integration of numerous techniques and methods from many areas to solve the difficulties and restrictions of implementing DRL in real-world scenarios. These situations frequently entail dynamic and complicated environments, where pure DRL techniques may be hindered by problems like sample inefficiency, security challenges, and high-dimensional state spaces. The goal of hybrid techniques is to improve performance, stability, and dependability by combining the benefits of DRL with those of other systems.
Скачать Deep Reinforcement Learning and Its Industrial Use Cases: AI for Real-World Applications

[related-news] [/related-news]
Комментарии 0
Комментариев пока нет. Стань первым!