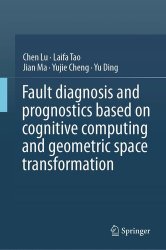
Автор: Chen Lu, Laifa Tao, Jian Ma, Yujie Cheng, Yu Ding
Издательство: Springer
Год: 2024
Страниц: 565
Язык: английский
Формат: pdf (true)
Размер: 47.8 MB
This monograph introduces readers to new theories and methods applying cognitive computing and geometric space transformation to the field of fault diagnosis and prognostics. It summarizes the basic concepts and technical aspects of fault diagnosis and prognostics technology. Existing bottleneck problems are examined, and the advantages of applying cognitive computing and geometric space transformation are explained. In turn, the book highlights fault diagnosis, prognostic, and health assessment technologies based on cognitive computing methods, including Deep Learning (DL), Transfer Learning, visual cognition, and compressed sensing. Lastly, it covers technologies based on differential geometry, space transformation, and pattern recognition.
For the emerging Deep Learning methods in the field of machine learning, the data features can be learned from raw data through a set of intelligent multilayer learning mechanisms to realize the accurate fitting of complex nonlinear functional relations. As to the autonomous learning of features for Deep Learning, the first thing is the learning mechanism based on a multilevel representation, which is obtained through the hierarchical combination of several simple nonlinear modules. After each layer of nonlinear transformation, the data can be expressed abstractly at a higher level. Through this multilevel abstract expression, the effective fitting of complex functional relations can be realized. Second, based on a learning mechanism that autonomously reduces the fitting errors, the learned features can be constantly adjusted, and hidden nonlinear features in the data can be gradually discovered.
Machine Learning (ML) is an important research direction of cognitive computing technology. From the hierarchical structures of Machine Learning models, the development of Machine Learning has generally undergone two waves of evolution, namely, shallow learning and Deep Learning, of which the latter is a new learning method emerged to overcome the limitations of shallow learning in the era of massive data. Compared with shallow structures, more complex, powerful, and deeper models can reveal the intrinsic information features and correlations carried by massive data, and make more accurate predictions about future or unknown events.
Скачать Fault Diagnosis and Prognostics Based on Cognitive Computing and Geometric Space Transformation

[related-news] [/related-news]
Комментарии 0
Комментариев пока нет. Стань первым!