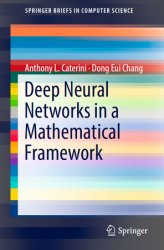
Автор: Anthony L. Caterini, Dong Eui Chang
Издательство: Springer
Год: 2018
ISBN: 9783319753041
Серия: SpringerBriefs in Computer Science
Формат: epub, pdf
Страниц: 84
Размер: 5,6 mb
Язык: English
The authors provide tools to represent and describe neural networks, casting previous results in the field in a more natural light. In particular, the authors derive gradient descent algorithms in a unified way for several neural network structures, including multilayer perceptrons, convolutional neural networks, deep autoencoders and recurrent neural networks. Furthermore, the authors developed framework is both more concise and mathematically intuitive than previous representations of neural networks.
This SpringerBrief is one step towards unlocking the black box of Deep Learning. The authors believe that this framework will help catalyze further discoveries regarding the mathematical properties of neural networks.This SpringerBrief is accessible not only to researchers, professionals and students working and studying in the field of deep learning, but also to those outside of the neutral network community.

[related-news] [/related-news]
Комментарии 0
Комментариев пока нет. Стань первым!