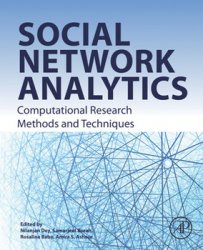
Автор: Nilanjan Dey, Samarjeet Borah
Издательство: Academic Press
Год: 2019
Страниц: 276
Язык: английский
Формат: pdf (true)
Размер: 29.6 MB
Social Network Analytics: Computational Research Methods and Techniques focuses on various technical concepts and aspects of social network analysis. The book features the latest developments and findings in this emerging area of research. In addition, it includes a variety of applications from several domains, such as scientific research, and the business and industrial sectors. The technical aspects of analysis are covered in detail, including visualizing and modeling, network theory, mathematical models, the big data analytics of social networks, multidimensional scaling, and more.
Sentiment analysis is the area which deals with judgments, responses as well as feelings, which is generated from texts, being extensively used in fields like data mining, web mining, and social media analytics because sentiments are the most essential characteristics to judge the human behavior. This particular field is creating ripples in both research and industrial societies. Generally there are different ways of classifying sentiments, the Machine learning approach and the lexicon-based approach being famous. When the Machine Learning approach is considered, it can be further categorized into supervised and unsupervised learning. While supervised learning can be defined as the process of learning from already known data to generate initially a model and further predict target class for the particular data, unsupervised learning can be defined as the process of learning from unlabeled to discriminate the provided input data.
Deep learning is considered to be a major part of Machine Learning which is mainly supported on methods and actions formalized to gain knowledge about multiple levels of feature depiction. Learning representations makes it easy to construct classifiers and predictors. The very latest applications that are being unearthed in the fields of deep learning are primarily in Automatic Speech Recognition, Image Recognition, Bioinformatics and also in Big Data Analytics, where features of Deep Learning were recognized which makes an impact on the analysis made in Big Data.
De-Anonymization Techniques for Social Networks is a process which when applied to an anonymized social network is likely to provide the original network as close as possible. It has two consequences. First, it may help the adversaries to get back the original networks so that a negative effect is attained, which is undesirable but unavoidable. Second, it will help the researchers who are developing anonymization algorithms to test the efficiency of their algorithms and make them more foolproof. We find that some of the researchers who have developed de-anonymization algorithms have claimed that their algorithms can de-anonymize any anonymized social network obtained as a result of any anonymization algorithm developed till that time.
As analyzing social network data is rapidly gaining interest in the scientific research community because of the importance of the information and insights that can be culled from the wealth of data inherent in the various aspects of the network, this book provides insights on measuring the relationships and flows between people, groups, organizations, computers, URLs, and more.
- Examines a variety of data analytic techniques that can be applied to social networks
- Discusses various methods of visualizing, modeling and tracking network patterns, organization, growth and change
- Covers the most recent research on social network analysis and includes applications to a number of domains
Скачать Social Network Analytics : Computational Research Methods and Techniques

[related-news] [/related-news]
Комментарии 0
Комментариев пока нет. Стань первым!