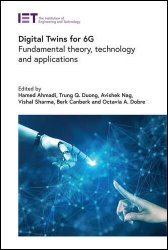
Автор: Hamed Ahmadi, Trung Q. Duong, Avishek Nag, Vishal Sharma, Berk Canberk, Octavia A. Dobre
Издательство: The Institution of Engineering and Technology
Год: 2024
Страниц: 341
Язык: английский
Формат: pdf (true)
Размер: 24.0 MB
Digital Twin (DT) technology is a real-time evolving digital duplicate of a physical object or process that contains all its history. It is enabled by massive real-time multi-source data collection and analysis. While 6G is considered as an enabler of digital twins, DT can also be a facilitator for integrating AI and 6G towards reliable, pervasive and efficient intelligent technologies. While the DT concept is familiar among aerospace and industrial engineers, it is a relatively new topic among electronic, electrical, computer, communications and networking engineers. For future massive-scale industrial internet-of-things (IoT) applications facilitated by DTs, a 6G network will be much more advantageous than its 5G counterpart. Digital Twins for 6G: Fundamental theory, technology and applications aims to bring together knowledge from industrial practitioners and researchers, and to introduce novel concepts that can help address the challenges associated with this interdisciplinary topic. The authors will cover fundamentals, enabling technologies, standards and advanced topics of DT and 6G to demystify the DT concept and its networking requirements and benefits, support a broader understanding of DT and its relationship with 6G to a larger audience, support learning and understanding for researchers and professionals working on 5G and 6G, and create a foundation on DT and 6G for the international research community. This book is intended to be both a tutorial of the important topics around digital twin and advanced wireless communications technologies, including 6G, as well as an advanced overview for technical professionals in the communications industry, technical managers, and researchers in both academia and industry.