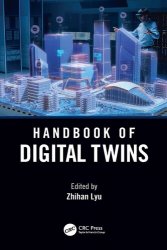
Автор: Zhihan Lyu
Издательство: CRC Press
Год: 2024
Страниц: 923
Язык: английский
Формат: pdf (true)
Размер: 13.5 MB
Over the last two decades, Digital Twins (DTs) have become the intelligent representation of future development in industrial production and daily life. Consisting of over 50 chapters by more than 100 contributors, this comprehensive handbook explains the concept, architecture, design specification and application scenarios of DTs.
As a virtual model of a process, product or service to pair the virtual and physical worlds, DTs allow data analysis and system monitoring by using simulations. The fast-growing technology has been widely studied and developed in recent years. Featured with centralization, integrity and dynamics, it is cost-effective to drive innovation and performance. Many fields saw the adaptation and implementation across industrial production, healthcare, smart city, transportation and logistics. World-famous enterprises such as Siemens, Tesla, ANSYS and General Electric have built smart factories and pioneered digital production, heading towards Industry 4.0.
The Cognitive Digital Twins (CDT) for manufacturing systems was proposed based on advances in cognitive science, Artificial Intelligence (AI) technologies, and Machine Learning techniques. According to the fundamental aspects of cognition, they define CDT as a DT with additional cognitive capabilities, including perception, attention, memory, reasoning, problem‑solving and learning.
Recall that a Digital Twin (DT) is a virtual copy of a physical asset that promptly reproduces and alters the state and behavior of the asset. DT intensely employs Industry 4.0 data‑driven Artificial Intelligence technologies and simulations for adaptive monitoring, forecasting, optimization, and controlling asset performance. The most accurate is the DT initiated at the beginning of the asset lifecycle, developed incrementally together with the asset, and put into operation synchronously with it. Computer‑aided design (CAD) tools apply to create a DT at the design stage, long before the asset comes into existence. Such an early DT is useful as a virtual testbed for evaluating alternative design decisions and choosing the best one. Moreover, the initial DT is synthesized fully automatically when advanced artificial intelligence technologies, known as generative design, are used.
Federated analytics can be an effective way for collaborative decision‑making. Federated analytics is proposed by the Google AI team, which allows data scientists to derive analytical insights among distributed datasets without collecting the data in a centralized server. Actually, federated analytics follows the framework of Federated Learning (FL), obtaining global aggregated results from uploads of individuals. The difference is that federated analysis only focuses on basic data science needs, such as model evaluation, data quality assessment, and heavyweight discoverers, and there is no learning part. Intuitively, there are several reasons to apply federated analytics for digital twins applications.
• Firstly, the digital twin is made up of two parts: physical objects or process that generates data and a virtual server that performs computation and makes decisions. Therefore, regarding a federated analytics problem, individual devices can be regarded as physical objects in digital twin and aggregator can be regarded as virtual server of the digital twin.
• Secondly, in federated analytics, what is transmitted within digital twins is the device’s individual analytical result instead of raw data. As a result, the communication latency can be decreased further.
• Thirdly, devices may be privacy‑sensitive, especially for healthcare and personal applications. In federated analytics, since data will not be shared within digital twins, the users’ privacy can be guaranteed as well.
From the perspective of data science, obtaining the distribution of the whole dataset based on evidence is important. However, because of the limitation of sensor range and mobility of connected devices, the generated individual datasets are only observed from a part of the entire data, i.e., individual datasets are not independent and identically distributed (non‑i.i.d.), which makes it hard to estimate the global distribution with the data from one device. Intuitively, the distribution of the dataset can be characterized by distribution parameters, like mean and variance for Gaussian distribution. Therefore, once the parameters can be evaluated, the global data distribution can be obtained accordingly. Fortunately, Bayesian approaches have been proved to be an effective method to tackle global parameter and posterior estimation‑related problems. The Bayesian approach estimates an unknown parameter by minimizing the posterior probability from all observed evidence. One typical method is the Monte Carlo method, which evaluates and approximates target distributions with random sampling techniques. The drawback of the Monte Carlo method is that the scheme heavily relies on the large volume repeating sampling. Therefore, how to conduct efficient sampling and guarantee the quality of samples at the same time becomes challenging.
This book aims to provide an in-depth understanding and reference of DTs to technical personnel in the field, students and scholars of related majors, and general readers interested in intelligent industrial manufacturing.
Скачать Handbook of Digital Twins

[related-news] [/related-news]
Комментарии 0
Комментариев пока нет. Стань первым!